Overview
Good engineering is an essential part of every research group in synthetic biology. However, we can not design and predict living organisms like architecture designs exact buildings, or aerospace engineers predict stars. So, other than top-down and bottom-up, biology scientists usually use “middle-out” approach to do the engineering. We, here, also introduce a testing-learning cycle to help us to check the result. Here shows the diagram of our engineering cycle.

The main purpose of our project is to create a stable enzyme that can decompose the high crystalline PET. We first investigate the PET enzyme. And then do research and interviews to get more information about it, to check out what is known and what is unknown. We found that the wild type PETase can degrade PET 5.5 times more efficiently than LCC but the thermal stability is quite bad. It is not able to survived under 40°C for 24h. Accordingly, we aimed to increase its thermostability. We made assumptions and built up models according to the information we got during investigating. The knowledge we learnt from classes and books also helped a lot. We tested those models and improved them by doing further research and learning from the result we got. Keep doing the testing-learning cycles can help us to reach the final success in engineering.
Doing Investigation
At the very beginning, we read some literature to decide what enzyme should be chosen to
use in our project. Then we found PETase, an enzyme that can degrade the high crystalline
PET. But PETase is not quite stable, nor effective. We found that the structure of it has
been resolved and published. There were some research groups tried to improve the stability
already, by different re-designing strategy. But none of them gets a satisfactory result.
We read the papers published by those research groups who wanted to improve the stability of
PETase and learnt from them. Also, we did more reading and consulted some experts in
bio-engineering to study how to change the features of proteins and enzymes.
Making assumptions
We applied the methods and principles, provided by the literature and the experts, such as adding hydrogen bonds, salt bridges, enhancing hydrophobic interactions, proline mutations and adding disulfide bonds, when making assumptions.
1st iteration
Modelling
Modelling is important in synthetic biology. A successful model can guarantee the success in implementation, the summary and feedback of the project. Here we used FoldX, SWISS-MODEL and other software to build up those models of the mutants we designed. It really helps us with the test of the stability of these mutants. Our group considered combining the sites with the best single point effect and combining the structure for analysis.
Testing
- ddG-Testing
- Structure verification
- Lab-Testing
FoldX, MD, Docking, PyMOL, Fireprot, CUPSAT, I-Mutant, etc. were used to predict the thermal stability of mutants. ddG, which is an important parameter to evaluate protein thermal stability was determined as well. Some of these methods gave the value of ddG of the protein directly, and others gave the instability index. We also turned ddG to dTm which characterized the thermal stability of the protein.
After making thermostability predictions, we used AlphaFold to predict the structures. We found that the structure of some single point mutations met our expectations, but many other mimicking models were not good enough. We found that the modeling structure of these 20 mutants is different from what we expected.
We collected 20 mutants, which act the best in this computer simulation, to go to the lab and tried to test their exact performance. We used affinity chromatography and gel filtration purification methods to separate and purify these mutant proteins, and use SDS-PAGE to verify the purity of the purified protein. All of these exist as inclusion bodies.
- We found that the modeling structure of these 20 mutants is different from what we expected.
- All of these exist as inclusion bodies. So, we failed to test the exact performance and get more information about them.
Learning & Improving
The function of different mutations may influence one another to give a better result. That’s what we wanted. But unfortunately we found that the increase of mutation sites may can silent the single point mutations. Things may act differently as the general theories suggested, nor the result of simulation experiments, especially in biology. So, we need more practice and find a better way to design the protein. We collected the differences between the real proteins and the simulated mutants and we got help from the group of University Toronto. We finally found the rule in the combination of different kinds of mutations. We also summed up the influence of the distance between different mutation sites. Accordingly, We carried out a redesigned combination of the single mutations
2nd iteration
Researching and Modelling
- After investigating the ways that can improve the expression of proteins, we decide to use different vectors that carry hydrophilic tags, which can auxiliary dissolvingauxiliary dissolving.
- We carried out a redesigned combination of the single mutations according to the rules we summarized from the first interation. We still used FoldX, Fireprot, CUPSAT, I-Mutant as simulating software to build up those models of the mutants we designed.
Testing
We still used the same way to predict the thermal stability of mutants and the same way to test the performance of these proteins. But we added a model of the relationship between UV260 and HPLC to simplify the test of performance according to some literature. We finally got some great ones with much higher thermostability.- We got the mutant number 20 (namely super4) as a great result, the optimal temperature of which is 50°C.
Learning & Improving
After we made this mutant with a degree of 50 degrees, we calculated all the 11 positions of it, and then found that one of the points, A80V, had not very good data, so we decided to replace this point with another mutation. To see if it can rise to a higher temperature, we have replaced six other combinations
3rd iteration
Modelling
After finding the problematic sites through calculations, we simulated six possible combinations which have similar mutation sites with mutant number 20 except A80V.
Test & Result
We still used the same method for testing and obtained very satisfactory results. The maximum optimum temperature of our mutant has reached 60 degrees and has a 163-Times higher degradation.
- We got the mutant number 24 (namely super5) as a great result, the optimal temperature of which is 60°C.
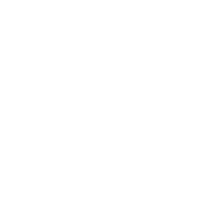