
Improvement of Parts
We designed 26 sequences in total and 7 of that encode the modified PETase with high thermostability(BBa_K3715005, BBa_K3715006, BBa_K3715001, BBa_K3715002, BBa_K3715003, BBa_K3715004, BBa_K3715007 )
PETase is an enzyme discovered in 2016 by Dr Kohei Oda, which can degrade the highly crystallized PET. The ability for wild type PETase to degrade PET film is relatively high, which is 5.5 times higher the wild type LCC. Many groups tended to improve the performance of PETase, including the decomposing ability, thermostability, secretion amount, etc. And there are also many groups in iGEM competition use PETase as a basic part of their design. Here, we provide a better choice for all these groups.
Super (BBa_K3715005) is the best result we got, the performance of which have risen by 163 times(measured by the amount of final product, MHET). It can survived under 65°C which is the highest temperature ever. Also, it gets its best performance at 60°C
It can be a better part for all the research groups who want to employ PETase as a part of their work. For example, if the group want to link LCC with PET together, to build a new enzyme which can degrade PET no matter high or low crystalline at the same time, they can use the Super 5 PETase. Thus, they don’t need to worry about the differences in Tm of PETase and LCC, so they can build an enzyme with better performance.If the group want to secret PETase as a reporter, they can use Super 5 as well. Because as the performance became better, the sensitivity can be higher.
Rule to stabilize protein
We recorded the experiment performance of all the enzymes we built in the whole project and found a rule between different mutations and the final stability of PETase.The rule will help all teams who want to modify the structure of the protein to improve the stability to reach their goal.
- The function of hydrophobic interaction is more predictable than any other intermolecular force.
- The hydrophobic interaction on loop area will help stable the structure but do not damage the flexibility dramatically.
- Adding hydrogen bonds or salt bridges in the same area on surface can enhance this stabilizing effect.
Contribution on tools
We set up our protocol for testing the performance of a catabolic enzyme.
- We first screened the decomposing efficiency of the enzyme after culturing it under 40°C for 18 h to get the proteins that maintain high efficiency.
- Then the optimum temperatures were determined by testing the enzyme performance under higher temperatures.
- We then prolonged the culture time to test the thermostability of the enzyme and used DSC to determine the melting temperature to test the thermal stability.
- Finally, SEM was used to visualize the degradation ability for PET of the enzyme.
Establish methods to accurately predict protein structure
In the choice of protein prediction methods, we have the choice of Alphafold2 and Alphafold, both of which are very good methods. Compared with Alphafold, alphafold2 has the characteristics of faster prediction speed and more accurate prediction results. During the operation of alphafold2, the target amino acid sequence, MSA, and template are required as input, and the 3D structure of the protein can be obtained "end-to-end". The specific prediction method mainly includes two parts of the neural network EvoFormer and the structure module (Structure module) Finally, the molecular docking process Autodock obtains the docking results and then screens the hit rate and active pockets to select the best docking result
Establishment of the conversion model between UV260 and HPLC Peak Area
UV absorbance at a certain wavelength of a compound is easy to be detected and can be determined accurately. UV absorbance at 260nm can detect the quantity of PET degradation products,and then achieve the measurement of PET degradation efficiency. HPLC can also provide accurate data about concentration but it needs a long time and complex operation. Also, HPLC cost a lot of money for every detection. We know the Beer-Lambert Law, the content of which reminded us of the linear relationship between concentration and UV absorption at a certain wavelength. We collected the data and set a calibration curve for further experiments to determine the concentration easily.
We conducted a lot of experiments to obtain the data needed to establish the functional relationship. In the choice of experimental data processing methods, we tried a variety of experimental methods including least squares, machine learning, and small neural networks. According to the amount of data obtained in the actual experiment, compared with the large demand for the amount of original data of the latter two, here we found that the least squares method is the best method to fit the data.
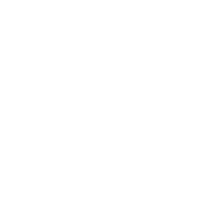